Prof. Nikos Melanitis from the Hellenic Naval Academy presents the NAVMAT platform, the knowledge-based system for the support of decision making and knowledge management of naval materials failures, at the 10th International Conference on Structural Analysis of Advanced Materials, 10-14 September 2023, on the island of Zante (Zakynthos), Greece.
TITLE
Self learning materials failure management system
AUTHORS
N. Melanitis 1, P. Giannaris 1, G. Giannakopoulos 2, K. Stamatakis 2, A. Sideras 2, D. Mouzakis 3, G. Melagraki 3, A. Koutsomichalis 4
1 Hellenic Naval Academy, Piraeus, Greece
2 Institute of Informatics & Telecommunications, NCSR Demokritos, Athens, Greece
3 Hellenic Army Academy
4 Hellenic Air-Force Academy
ABSTRACT
The H.F.R.I. funded project (no 822) with the acronym NAVMAT intends to develop a knowledge-based system for the support of decision making and knowledge management of naval materials (NAVMAT) failures. The work aims mainly to erase disruptions in the flow of knowledge in a versatile environment such as a fleet, to support the decision making in maintenance and supply of marine spares, to train and educate the engineers and the operators of an organisation with distributed unis and points of presence, to contribute to operational readiness, by developing a materials failure ontology and utilising artificial intelligence algorithms in data handling [1].
The key data of the system is the failure incidents. Each one is associated with a component, which belongs to a system of a naval (and not only) platform. Once the failure incident is recorded in NAVMAT system by the authorized and trained users, should a failure analysis be commissioned, is then associated with a failure mode, and a root cause of failure, together with other characteristics of the material and the conditions of operation [2].
The NAVMAT application supports several types of users, from the incident contributor to the materials expert through appropriate workflows. Multiple types (and language) of information are incorporated securely to the system such as text, files, images, and videos. They are classified by using a dedicated materials, components and systems failure ontology developed, and subsequently indexed and retrieved upon request by employing Artificial Intelligence and machine learning tools.
The prototype application is developed in a web environment and has been fed with 40 failure incidents and associated failure analysis reports. A User Group of 30 volunteer experts has been formed and thoroughly examined the system, reported bugs, and provided suggestions to improve the user experience of the application. The application is expected to be fully operational by the end of the current year.
REFERENCES
1. Nagaraja Rao, B. K., The Role of Artificial Intelligence (AI) in Condition Monitoring and Diagnostic Engineering Management (COMADEM): A Literature Survey. American Journal of Artificial Intelligence. Vol. 5, No. 1, pp. 17-37 (2021)
2. N. Melanitis, G. Giannakopoulos, K. Stamatakis, D. Mouzakis, A. Koutsomichalis, Designing a knowledge management system for Naval Materials Failures, ICEAF-VI 2021, MATEC Web of Conference 349, 03006 (2021)
WEBSITE
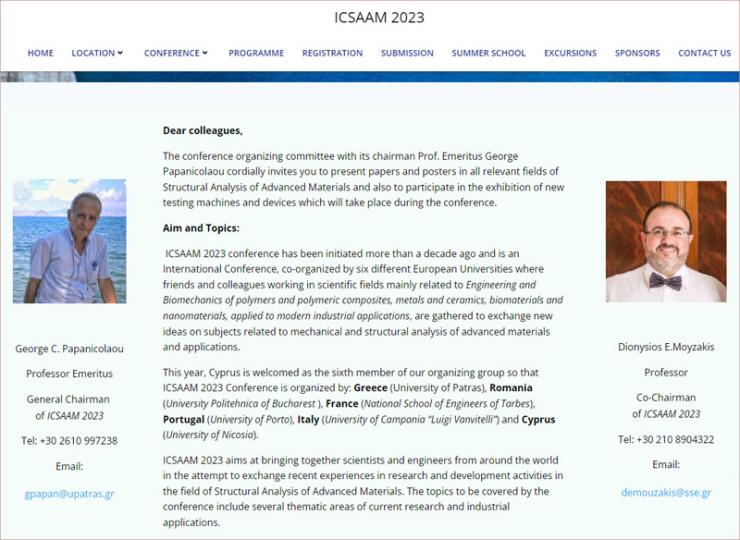